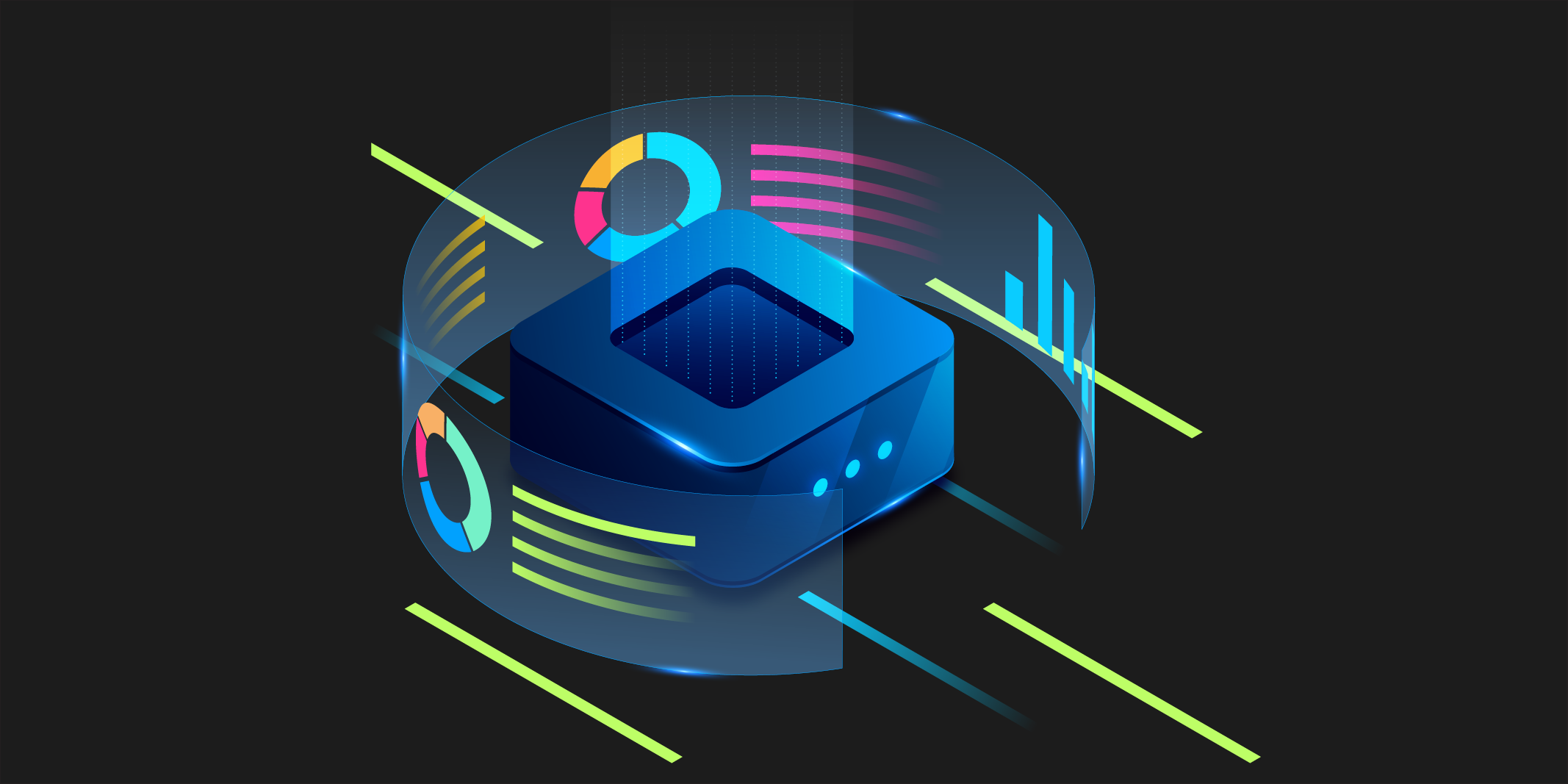
As artificial intelligence (AI) has developed, many have hailed synthetic data as a promising answer to the problems of privacy issues and data scarcity.Theoretically, training large language models (LLMs) on synthetic data would enable training sets to grow quickly without requiring the expensive human datacollection. Research on regurgitative training and recent work from Stanford's Human-Centered AI group, among other studies, suggests that this strategy has limitations.
The Drawbacks of Artificial Data: Model Failure
Model collapse is a recurrent theme, which occurs when models trained solely on synthetic data progressively lose the diversity present in real-world inputs. For example, the 2024 AI Index Report by Stanford highlights how relying solely on synthetic augmentation causes models to “forget” the rare, but important, details present inhuman-generated data. In one study, researchers observed that as synthetic data was recycled over generations, the outputs became increasingly centered around the mean, effectively losing the “tails” of the distribution that capture minority or edge cases. Quantitatively, experiments have shown that metrics such as lexical diversity decrease with each synthetic iteration, leading to a measurable degradation in performance.
Human Input: The Base and Ongoing Quality Check
Even in models where synthetic data is used to balance human data, the process for training models has to start, and most of the time will continue, with well-curated human-generated inputs. Research in regurgitative training (the practice of training new models on data generated by previous models) finds that even when they include synthetic examples, a very small ratio of mixing in human data can prevent degradation in performance. A task of machine translation, for example, showed substantial improvements in BLEU scores when just a tiny fraction of real data was included in the training, as against purely synthetic outputs (arxiv.org).
Furthermore, human intelligence remains crucial in evaluating the quality and output of synthetic candidates. Automated metrics can capture performance aspects, but subtle errors concerning hallucinations and decreased diversity can be detected only by human evaluators in particular contexts and with some common sense. The fact that a leading AI lab is employing reinforcement learning from human feedback (RLHF)is a manifestation of this point. By having human annotators rank model outputs, systems can be piloted where correctness rather than creativity and nuance is prioritized.
Detail Case Studies with Quantitative and Qualitative Metrics
To strengthen the reasoning, consider the case studies given below. The following two should provide illustrative cases:
1) Machine Translation and BLEU Scores: Recently, researchers fine-tuned GPT-3.5 on three types of data-pure synthetic, mixed, and purely human-generated. Quantitatively, those trained solely on synthetic yielded a drop of over 15% in BLEU linear scores as opposed to their real-trained counterparts. Qualitatively, the outputs were reported to be less diverse and error-prone, indicating that those variables alone do not sustain high-quality language generation over time.
2) Image Generation and Diversity Metrics: Similar trends have been observed in generative imaging models. When synthetic data is used in training loops without human intervention, the FID (Fréchet Inception Distance) scores tend to worsen over successive generations. Detailed evaluations revealed that the quality improvement seen with synthetic augmentation quickly plateaued, reinforcing the need for an infusion of human-generated images to maintain diversity.
Recommendations for Future AI Development
The following key recommendations are recommended to practitioners and researchers:
Blend, don’t replace: Make use of synthetic data along with quality human-generated data. Evidence from various exhaustive works proposes that even a small amount may stay this verity of model collapse.
Invest in evaluation: Combine automated evaluation metrics and human verification for giving credit to the system used in evaluation. Extend other form of quantitative evaluation such as BLEU and FID scores with some qualitative assessment of work done by actual experts.
Document and share metrics: For full transparency and reproducibility, publish both quantitative (error rates and diversity metrics) and qualitative assessments (expert reviews) of data quality. This will help the community to better understand the value and limitations of synthetic data.
Also Read: Why Apex’s Managed Workforce Model Outshines Crowdsourcing in AI Projects
Conclusion
While synthetic data offers a promising way to alleviate data scarcity and preserve privacy, it cannot replace the nuanced, diverse, and high-quality information that only human-generated data can provide. As research continues to reveal, robust AI systems must be built on a solid foundation of real data—and maintained with careful, ongoing human oversight.
For organizations leveraging synthetic data, Apex Data Sciences offers specialized RLHF (Reinforcement Learning from Human Feedback) services to fine tune AI models.